Prof. Dr. Peter Grünwald
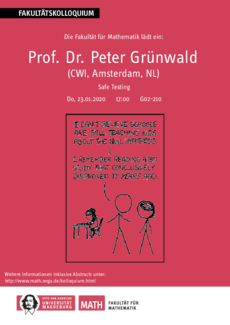
A large fraction (some claim > 1/2) of published research in top journals in applied sciences such as medicine and psychology is irreproduceable. In light of this 'replicability crisis', standard p-value based hypothesis testing has come under intense scrutiny. One of its many problems is the following: if our test result is promising but nonconclusive (say, p = 0.07) we cannot simply decide to gather a few more data points. While this practice is ubiquitous in science, it invalidates p-values and error guarantees.
Here we propose an alternative hypothesis testing methodology based on gambling (or in more mathematical terms: nonnegative supermartingales). This safe testing method allows us to consider additional data and freely combine results from different tests. While this idea is not new (it has been advocated e.g. by Vovk, one of Kolmogorov's students), in the past it could only be applied to problems with a 'simple' null hypothesis (no free parameters, e.g. testing whether a coin is fair). Yet nearly all tests used in practice, such as the t-test or independence tests, have nonsimple, 'composite' null hypotheses (i.e. free parameters). Based on a novel minimax theorem, we show that safe tests can be constructed for arbitrary composite testing scenarios as well. This allows us to formulate safe versions of some of the most popular tests used in practice.
We end the talk by briefly reviewing the three main paradigms of testing: Fisherian, Neymanian, and Bayesian. Even now, a hundred years after the inception of modern statistics, there is no consensus on which one is 'right'. It turns out that, unlike currently used tests, safe tests have a valid interpretation within all three paradigms.
Joint Work with R. de Heide (CWI and Leiden Univ.) and W. Koolen (CWI).
23.01.2020, Raum: G02-210, Zeit: 17:00